The dark art of gathering requirements
Gathering requirements for a data project is both painful and essential. But there are ways to make it easier…
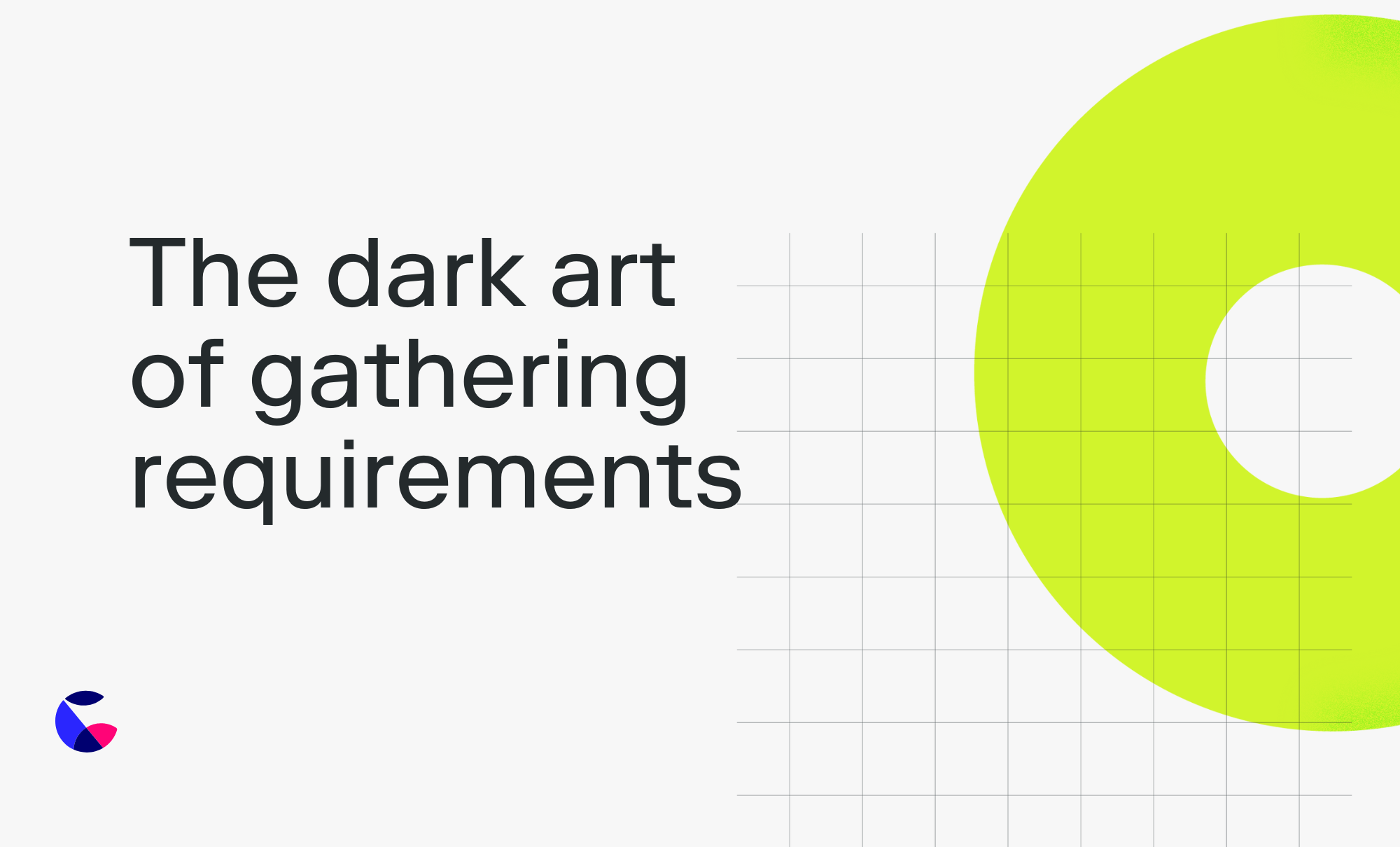
Like many people in the data community recently I’ve decided to join Mastodon to engage with the community on all things data. (Come find me at: https://data-folks.masto.host/@othhughes). Last week I decided to test out the platform’s polling feature to get some data on a topic I’ve been thinking about recently:- Why analytics often feels so inefficient?
Where does our time go?
Here’s the “Toot” (Mastodon’s equivalent to a tweet 😂):

Now this was far from the most scientific survey, but nevertheless, the answer was pretty definitive and validated my suspicion… we waste most of our time working on the wrong questions!
When you step back this isn’t surprising. A data project is effectively a process and if you put rubbish in at the beginning then you’re going to get rubbish out at the end. The other issues listed in the survey like data quality and dealing with stakeholder feedback are definitely pain points but when they happen they have localised impacts. Nothing has the potential to completely derail a data project like poorly defined objectives can.

Now all this talk of ambiguous scope and wasted work can be quite triggering for data analysts. I’ve heard so many horror stories of this happening; the deep dive analysis which took over a week from which the stakeholder only uses one chart, or the dashboard we built that never actually gets used. We’ve all been burned here and the hard thing to acknowledge is that we also know the solution, but it’s a bitter pill to swallow. Gathering requirements…
But first: Fighting ‘not-my-problem-ism’
Often when I speak to data people about this topic, I get the response that this isn’t a data team problem to solve. “It’s up to stakeholders to know what they want,” etc etc. And while stakeholders undeniably have a role to play in the solution, we must not ignore or diminish our own ability to change the way things are.
If this is indeed the most painful and prevalent part of doing analysis today, then we must acknowledge our own agency to change it. The recommendations below come from speaking to a range of different data teams and are small changes to our workflow that have the potential to make large improvements to how we can drive value for our organisation in ambiguous situations.
Slowing down to go faster
Benjamin Franklin is famous for saying: “By failing to plan, you are preparing to fail.” We know there’s a lot to be gained by defining the scope of a project upfront. Most data teams implement some sort of data request/ticketing system to collect some of these requirements upfront.
Pushing for more upfront clarity helps to avoid false starts and going down very unhelpful paths of investigation. There are also other side benefits of this upfront agreement. It allows data teams to:
- Understand the urgency of the request and understand the needs behind the request
- Manage expectations on what is possible
- Provide suggestions on ways to find a better answer (hopefully!)

What I’ve said so far shouldn’t be controversial. I don’t know anyone who wouldn’t look to clarify the scope of a larger analytical project like a new KPI report or data model ahead of time. But for a large number of requests, the ability to sit down and fully map out the work in perfect detail just feels inappropriate or even impossible.
Almost by definition some problems don’t have a clear line of investigation and only by doing the work does the solution or end result become clear. Other requests are so trivial that the time it takes to fully scope them would probably be longer than just doing the work.
So what do we do? Ambiguous projects come up regularly and, because they are focused on discovering something unknown, can produce significant business value. How do we dive into these projects whilst avoiding the headaches and wasted time we know they can cause?
Fortunately, there are other methods for creating and maintaining a clear scope of a data project that can either supplement or replace upfront scoping in certain situations...
Option 1: Map out the problem together
Analysts have an enormous wealth of knowledge about the data available to the business. When a project has hazy objectives it’s tempting to just dive in and “explore the data” and see if we can produce something which gives value. A less risky approach would be for data analysts to map out the problem space with the stakeholder - listing out the elements of the project which are understood, those that aren’t, and sharing what data is available to help bridge the gap between a business objective and what the data can show.
This project “dry run” could result in a few different outputs - it could be a wireframe of the final charts or report, or a list of questions that both the analyst and stakeholder agree would provide a definitive answer to the problem.
The other advantage of this approach is that by starting to solve the problem together both the stakeholder and analyst have increased clarity and buy-in on what the project is setting out to achieve. Who doesn’t want that?
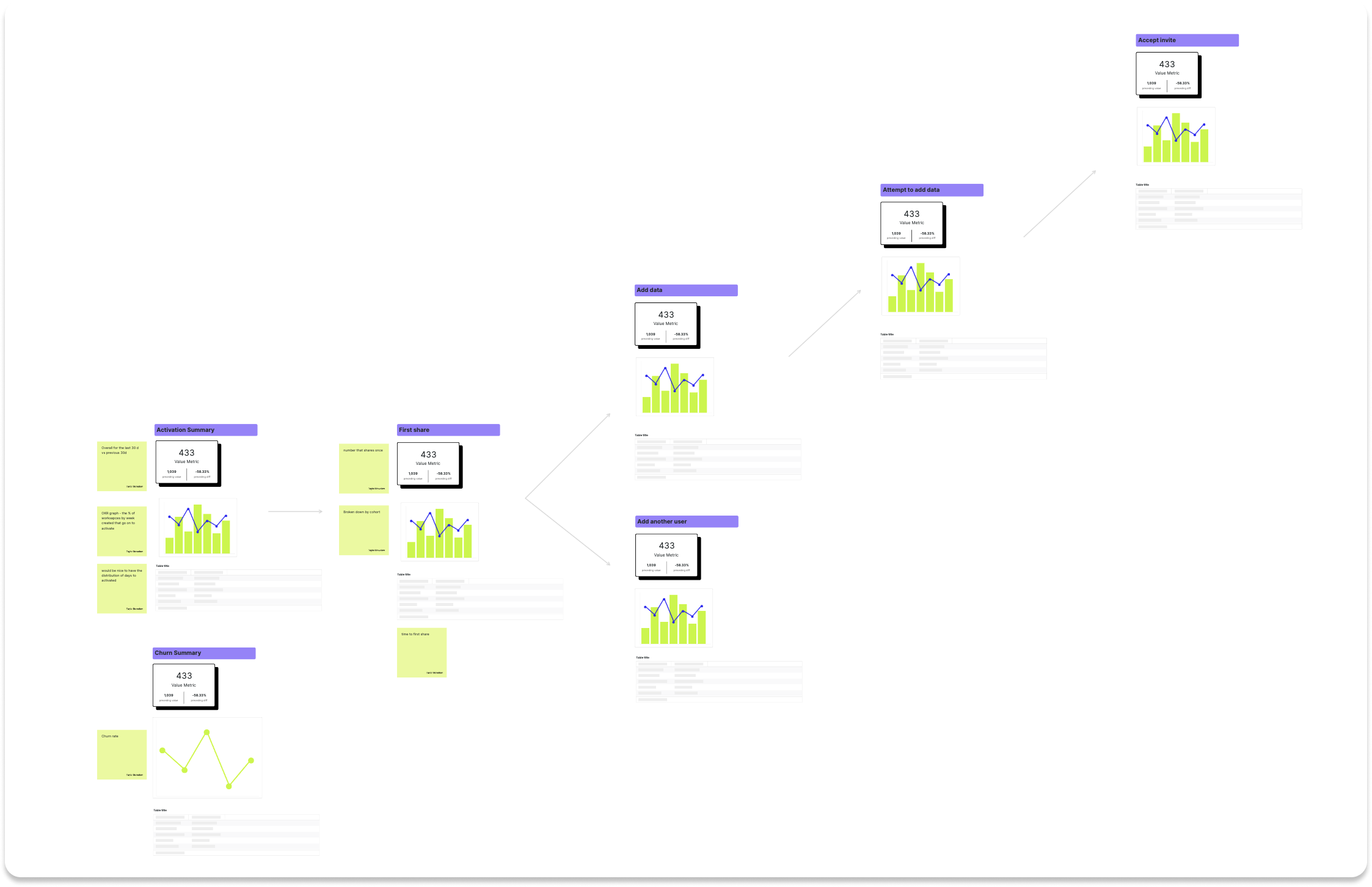
Option 2: Staying agile
Sometimes the end goal of a project is never going to be clear until the work has started. Again the big temptation in this situation is for the analyst to go away and do a big investigative analysis to see what they can find. Stakeholders love receiving lots of topical insights (it’s all “interesting”) but this is rarely the fastest way to solve the core issue.
A better approach is to agree on a “minimum viable analysis” where the analyst does the minimum amount of work they can to bring back some analysis in a pre-agreed area of investigation to discuss with the shareholder.
The goal is to keep the iteration loop as small as possible. This minimises the amount of overwork and allows the project to be tightly steered toward the most promising conclusion.
Sharing a half-finished analysis can feel odd at first but it has a few advantages:
- Builds trust in the results as they come no “grand reveal” moments which are high risk and often kick off lots of additional explanatory work
- Increases buy-in and validates continual interest in the project

Whaddaya know!? Communication is key
None of these approaches above are mutually exclusive, in fact blending all three is often the most effective. If you do some upfront scoping, refine the scope by mapping the problem then work iteratively towards a conclusion then it’s hard to go too far wrong.
The key principle behind all these approaches is that the better and more frequently the data team and business communicate, the better and more efficiently the project can be delivered.
We’ve seen teams using Count in this way reduce the time it takes to complete a data project by some shocking amounts by reducing initial briefs to what really matters and increasing the frequency of the feedback loop.
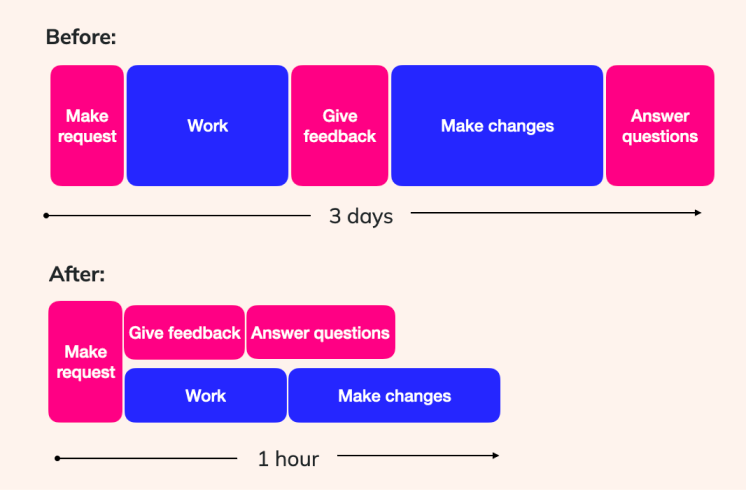
As mentioned above, this way of working has the benefit of changing the role that data teams play in the organisation. When the submission of a data request and the delivery of the analysis is transactional, data teams risk being perceived more and more as “chart factories”. By introducing more of a collaborative way of working data teams have the opportunity to position themselves as a core partner in solving an organisation’s biggest problems.
In the end, if you are one of the 66% of my Mastodon followers who struggle with constantly changing requirements, the answer is definitely not to give up. We can play a bigger role in the speed and impact of our work than we think. Now it’s up to us to change it.